Visual Perception
Learn the fundamentals object tracking, image segmentation, appearance matching, and neural networks for visual perception.
Modules/Weeks
Weekly Effort
Discipline
School
Format
Cost
Course Description
- Explore object tracking challenges. Dive into the intricacies of tracking objects within complex scenes, addressing challenges like change detection for object-background differentiation.
- Master video-based object tracking. Develop expertise in tracking objects within video sequences, considering factors like pose changes and occlusion effects.
- Hone image segmentation techniques. Delve into image segmentation intricacies, adopting a bottom-up approach that groups similar attribute pixels to form coherent regions.
- Conquer object recognition methods. Grasp two approaches to object recognition: direct recognition using appearance and dimension reduction techniques, and utilizing neural networks for mapping input images to object classes, identities, or activities.
Course Prerequisites
- Strong foundation in linear algebra and calculus
- Familiarity with any programming language will aid in understanding software implementation of course methods
- Prior experience in imaging or computer vision is not a prerequisite
What You Will Learn
By the end of this course, learners will be able to:
Design Advanced Scene Analysis Algorithms: Develop algorithms to detect significant changes within scenes, enabling the extraction of meaningful insights.
Master Object Tracking in Videos: Acquire proficiency in object tracking techniques across diverse video scenarios, accounting for varying conditions and challenges.
Excel in Image Segmentation: Employ multiple methodologies for segmenting images into coherent and significant regions, enhancing the comprehension of image content.
Build End-to-End Object Recognition Pipelines: Develop complete pipelines for learning and recognizing objects based on visual attributes, demonstrating the ability to create holistic solutions.
Demonstrate a Solid Grasp of Neural Networks and Backpropagation: A comprehensive understanding of neural networks and the backpropagation algorithm, empowering them to effectively apply machine learning principles.
Course Outline
Module 1: Introduction to first principles of computer vision
Module 2: Object tracking
Module 3: Image segmentation
Module 4: Appearance matching
Module 5: Neural networks
Instructors
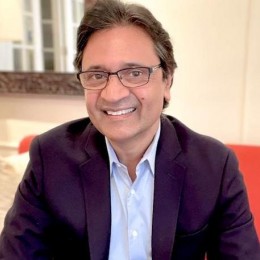
Shree K. Nayar is the T.C. Chang Professor of Computer Science at Columbia University and leads the Columbia Vision Laboratory. His research focuses on developing advanced computer vision systems for digital imaging, computer graphics, robotics, and human-computer interfaces. He has received numerous awards and honors for his research and teaching, including the David Marr Prize, the David and Lucile Packard Fellowship, and the National Young Investigator Award. Nayar has also been elected to the National Academy of Engineering, the American Academy of Arts and Sciences, and the National Academy of Inventors. He holds a BS degree in Electrical Engineering from Birla Institute of Technology, an MS degree in Electrical and Computer Engineering from North Carolina State University, and a PhD degree in Electrical and Computer Engineering from the Robotics Institute at Carnegie Mellon University.
Please note that there are no instructors or course assistants actively monitoring this course.