Term-Structure and Credit Derivatives
Learn about interest rate evolution, credit derivatives, fixed income, model calibration, securitization, and Collateralized Mortgage Obligations.
Modules/Weeks
Weekly Effort
Discipline
School
Format
Cost
Course Description
- Analyze the evolution of interest rates and gain insights into credit derivatives.
- Examine term structure lattice models and evaluate fixed income derivatives, including options, futures, caplets, floorlets, swaps, and swaptions.
- Apply model calibration techniques to fixed income securities and extend them to other asset classes and instruments, using Excel to price a payer swaption in a Black-Derman-Toy (BDT) model.
- Introduce and focus on modeling and pricing Credit Default Swaps, followed by an overview of asset-backed securities (ABS), Mortgage-Backed Securities (MBS), and Collateralized Mortgage Obligations (CMOs) pricing.
Course Prerequisites
- Intermediate to advanced undergraduate courses in probability, statistics, linear algebra, calculus, and optimization
- Programming questions can be completed using Excel and Python or other preferred languages
- Prior exposure to introductory finance, including understanding interest rates, discounting, compounding, and basic familiarity with options and futures
- Recommended to start with "Intro to Financial Engineering and Risk Management" for a comprehensive learning experience and preparation for academic and industrial problem-solving.
What You Will Learn
By the end of this course, learners will be able to:
Demonstrate a profound understanding of valuing complex financial derivatives using stochastic models.
Apply a systematic and data-driven approach to formulate modeled returns and risks for various asset classes and optimal portfolios.
Backtest and implement trading models and signals in an active, live trading environment.
Navigate and excel in the rapidly-evolving field of finance and quantitative trading.
Course Outline
Module 1: Course introduction
Module 2: Term structure models I
Module 3: Term structure models II (and Introduction to credit derivatives)
Module 4: Introduction to credit derivatives
Module 5: Introduction to mortgage mathematics and mortgage-backed securities
Module 6: Assignment - CMO
Instructors
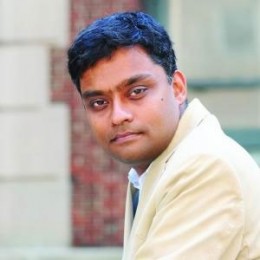
Garud Iyengar joined Columbia University’s Industrial Engineering and Operations Research Department in 1998 and teaches courses in asset allocation, asset pricing, and simulation and optimization. His research interests include convex optimization, robust optimization, queuing networks, combinatorial optimization, mathematical and computational finance, and communication and information theory.
Professor Iyengar received a Ph.D. in Electrical Engineering from Stanford University. He also holds a Master of Science in Electrical Engineering from Stanford University and a Bachelor of Technology from the Indian Institute of Technology.
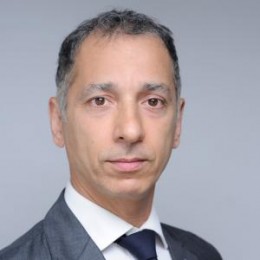
Professor Ali Hirsa joined IEOR in July 2017. He had been associated with Columbia University as an Adjunct Professor since 2000. He is also Managing Partner at Sauma Capital, LLC a New York Hedge Fund.
Previously Ali was Managing Director and Global Head of Quantitative Strategy at DV Trading, LLC, a Partner and Head of Analytical Trading Strategy at Caspian Capital Management, LLC. Prior to joining Caspian, he worked in a variety of quantitative positions at Morgan Stanley, Banc of America Securities, and Prudential Securities. Ali was also a Fellow at Courant Institute of New York University in the Mathematics of Finance Program from 2004 to 2014.
Ali’s research interests are algorithmic trading, machine learning, deep learning, data mining, optimization, computational and quantitative finance. His focus has been on machine learning applications in finance specifically in asset management and also developing learning algorithms on signal extraction from data.
Ali is author of “Computational Methods in Finance”, Chapman & Hall/CRC 2012 and co-author of “An Introduction to Mathematics of Financial Derivatives”, third edition, Academic Press and is the Co-Editor-in-Chief of Journal of Investment Strategies. He has several publications and is a frequent speaker at academic and practitioner conferences.
Ali is co-inventor of “Methods for Post Trade Allocation” (US Patent 8,799,146). The method focuses on allocation of filled orders (post-trade) on any security to multiple managed accounts which has to be fair and unbiased. Current existing methods lead to biases and the invention provides a solution to this problem.
He is currently member of Board of Visitors of College of Computer, Mathematical, and Natural Sciences at University of Maryland College Park since June 2016 and was on Board of Visitors at A. James Clark School of Engineering from 2008 to 2018 and served as a trustee on University of Maryland College Park Foundation from 2011 to 2016.
Ali received his PhD in Applied Mathematics from University of Maryland at College Park under the supervision of Professors Howard C. Elman and Dilip B. Madan.
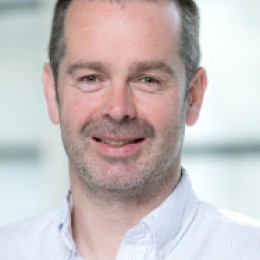
Martin Haugh joined Imperial College Business School as an Associate Professor in 2017. Prior to that he spent more than 10 years in the Department of IE & OR at Columbia University as well as 4 years working in the hedge fund industry in New York and London. He obtained his PhD in Operations Research from MIT in 2001 and has MS degrees in Mathematics and Applied Statistics from Cork and Oxford, respectively.
Prior to joining Imperial College, Professor Haugh was an Associate Professor of Practice at Columbia University.
Martin's main research interests are in: (1) Computational finance and risk management and (2) Markov Decision Processes and sub-optimal control. He is also very interested in: (3) Machine Learning / Business Analytics / “Big Data” and has recently begun working on some research projects in this area.
Please note that there are no instructors or course assistants actively monitoring this course.