Optimization Methods in Asset Management
Gain an understanding of optimization methods applied to portfolio construction and risk management, including additional information and techniques used in the topic.
Modules/Weeks
Weekly Effort
Discipline
School
Format
Cost
Course Description
- Explore portfolio construction using mean-variance analysis and capital asset pricing Model.
- Apply the security market line and Sharpe optimal portfolio in asset allocation.
- Examine risk measurements like value at risk and conditional value at risk.
- Enrich mean-variance portfolio strategies by considering transaction costs and exploring Exchange Traded Funds (ETFs) and transaction cost modeling.
Course Prerequisites
- Intermediate to advanced undergraduate courses in probability and statistics, linear algebra, calculus, and optimization
- Strong mathematical foundation to comprehend advanced topics in marketing analytics
- Foundational knowledge of marketing analytics
What You Will Learn
By the end of this course, learners will be able to:
Value complex financial derivatives, including options, swaps, forwards, and futures, using stochastic models.
Formulate modeled returns and risks for various asset classes and construct optimal portfolios in a systematic, data-driven approach.
Back test and implement trading models and signals in a live trading environment, enhancing their ability to make informed investment decisions.
Gain a strong understanding of financial modeling principles and apply this knowledge effectively in real-world investment scenarios.
Course Outline
Module 1: Course introduction
Module 2: Mean-variance analysis and CAPM
Module 3: Assignment week
Module 4: Practical issues in implementing mean variance
Module 5: Assignment week
Module 6: Other applications of financial engineering
Instructors
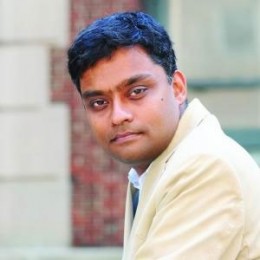
Dr. Garud Iyengar is the Tang Family Professor of Industrial Engineering and Operations Research and Senior Vice Dean of Research and Academic Programs. His research is dedicated to understanding uncertain systems and exploiting available information using data-driven control and optimization algorithms. Iyengar's research group has explored diverse fields such as machine learning, systemic risk, asset management, operations management, sports analytics, and biology. They are currently working on several projects, including thermodynamics of sensing and memory in cells, automatic defensive assignment and event detection in NBA games, and attribution schemes for allocating payment in multi-channel advertising. Additionally, they are working on systemic risk associated with extreme weather, a deep neural-network-based framework for interpretable robust decision making, and an NLP-based model for predicting stock performance using news reports. Iyengar received his B Tech in electrical engineering from the Indian Institute of Technology in 1993 and his PhD in electrical engineering from Stanford University in 1998. He is also a member of Columbia's Data Science Institute.
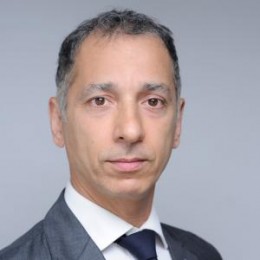
Professor Ali Hirsa joined IEOR as a faculty member in July 2017, having previously served as an Adjunct Professor at Columbia University since 2000. In addition to his academic work, he is also Managing Partner at Sauma Capital, LLC, a New York Hedge Fund. Prior to this, he held a range of quantitative positions at firms such as Morgan Stanley, Banc of America Securities, and Prudential Securities. His research focuses on machine learning applications in finance, asset management, signal extraction from data, and other areas of computational and quantitative finance. Ali is the author of several publications, including “Computational Methods in Finance” and “An Introduction to Mathematics of Financial Derivatives”. He is also the Co-Editor-in-Chief of Journal of Investment Strategies and has spoken at various academic and practitioner conferences. Ali is a co-inventor of the "Methods for Post Trade Allocation" patent, which aims to create a fair and unbiased method for allocating filled orders to multiple managed accounts. Ali received his PhD in Applied Mathematics from the University of Maryland at College Park.
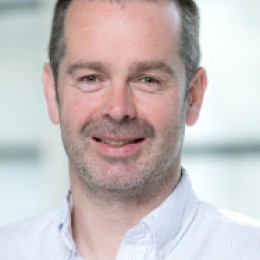
Martin Haugh is an Associate Professor at Imperial College Business School, having previously spent over a decade in the Department of IE & OR at Columbia University and four years in the hedge fund industry in New York and London. He holds a PhD in Operations Research from MIT and MS degrees in Mathematics and Applied Statistics from Cork and Oxford, respectively. Before joining Imperial College, he served as an Associate Professor of Practice at Columbia University. Martin's research focuses on computational finance and risk management, Markov Decision Processes, sub-optimal control, and machine learning/business analytics/"big data". He has recently begun working on research projects in the machine learning and business analytics space.
Please note that there are no instructors or course assistants actively monitoring this course.