Introduction to Financial Engineering and Risk Management
Learn the practical applications of financial engineering models, case studies, and industry practices, providing a foundation for further study.
Modules/Weeks
Weekly Effort
Discipline
School
Format
Cost
Course Description
- Apply simple stochastic models to price derivative securities across various asset classes.
- Analyze the role of different asset classes during the financial crisis and understand their impact.
- Solve real-world problems using financial engineering models and industry practices.
- Develop skills in derivatives pricing and modeling, building a strong foundation for further studies in Financial Engineering and Risk Management.
Course Prerequisites
- Completed intermediate to advanced undergraduate courses in probability and statistics, linear algebra, and calculus.
- The ability to complete course assignments using Excel and Python (preferred).
What You Will Learn
By the end of this course, learners will be able to:
Gain a comprehensive understanding of financial engineering and risk management concepts and practices.
Familiarize themselves with prerequisite concepts in probability and optimization, essential for grasping the mathematical fundamentals of the course.
Explore various topics, including fixed-income securities, derivative instruments, swaps and options pricing, option pricing in a multi-period setting, and the multi-period Binomial Model.
Apply their knowledge practically by working with examples that utilize the 1-period Binomial and the Black-Scholes Models, culminating in an assignment to demonstrate their proficiency.
Course Outline
Module 1: Course Introduction
Module 2: Prerequisite Materials
Module 3: Introduction to Basic Fixed Income Securities
Module 4: Introduction to Derivative Securities
Module 5: Option Pricing in the Multi-Period Binomial Model
Module 6: Assignment
Instructors
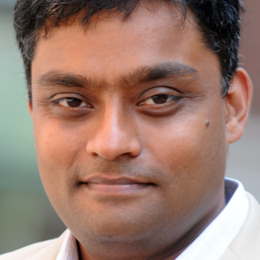
Dr. Garud Iyengar is the Tang Family Professor of Industrial Engineering and Operations Research and Senior Vice Dean of Research and Academic Programs. His research is dedicated to understanding uncertain systems and exploiting available information using data-driven control and optimization algorithms. Iyengar's research group has explored diverse fields such as machine learning, systemic risk, asset management, operations management, sports analytics, and biology. They are currently working on several projects, including thermodynamics of sensing and memory in cells, automatic defensive assignment and event detection in NBA games, and attribution schemes for allocating payment in multi-channel advertising. Additionally, they are working on systemic risk associated with extreme weather, a deep neural-network-based framework for interpretable robust decision making, and an NLP-based model for predicting stock performance using news reports. Iyengar received his B Tech in electrical engineering from the Indian Institute of Technology in 1993 and his PhD in electrical engineering from Stanford University in 1998. He is also a member of Columbia's Data Science Institute.
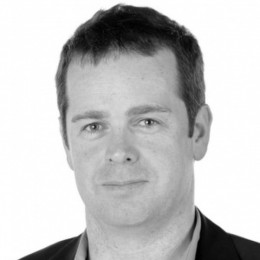
Dr. Martin Haugh is an Associate Professor at Imperial College Business School, having previously spent over a decade in the Department of IE & OR at Columbia University and four years in the hedge fund industry in New York and London. He holds a PhD in Operations Research from MIT and MS degrees in Mathematics and Applied Statistics from Cork and Oxford, respectively. Before joining Imperial College, he served as an Associate Professor of Practice at Columbia University. Martin's research focuses on computational finance and risk management, Markov Decision Processes, sub-optimal control, and machine learning/business analytics/"big data". He has recently begun working on research projects in the machine learning and business analytics space.
Please note that there are no instructors or course assistants actively monitoring this course.